By James McPhail
The commercial building sector represents 18% of all energy consumed in the United States. It not only represents a huge area of impact for the U.S., but for businesses, understanding the sector can represent an opportunity for improved economics and cutting costs. Given commercial buildings’ large scale, understanding its behavior as a group sheds insight into greater market trends and tips for individual commercial customers. For example, leveraging artificial intelligence (AI) can yield insight into energy pricing that customers and energy users can use to hedge resources and take advantage of key incentives.
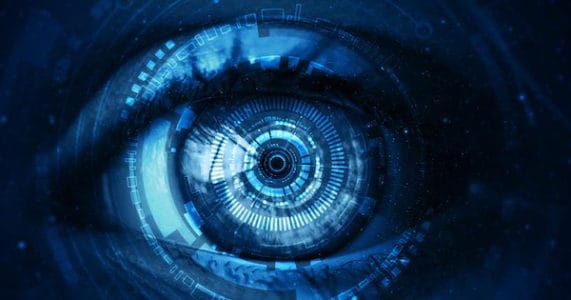
The Demand Management Value Stack
Demand management simply involves modifying energy-consuming behavior based on financial incentives or education. Today, two main financial mechanisms largely influence commercial customers’ behavior: they are peak demand charges and demand response. These financial mechanisms set simplistic business rules for companies to operate around and that software can facilitate.
The first, peak demand charges, represent a large part of commercial and industrial customers’ utility bills and, therefore, are often important drivers for demand management. With peak demand charges, every month or so, utilities charge commercial and industrial energy users based on the maximum amount of power they drew from the grid for a given interval of time, typically 15 minutes. Not knowing when these demand charges occur, commercial customers are generally incentivized to prevent their energy consumption from getting too big at any given time.
The second, demand response incentivizes customers to lower their electricity use in response to utility-driven signals. Utilities deploy near-real-time demand response signals for relief when system reliability is in jeopardy. Regions can also implement demand response through policy, such as California’s time-of-use rates that encourage electricity use during set off-peak demand hours by pricing the cost of electricity lower then.
Applying AI To Anticipate Peak Events
Online portals can give businesses management control of heating, ventilation and air conditioning (HVAC) systems and other energy devices while also identifying patterns and applying lessons learned from orchestrating energy savings at scale. For example, Zen Ecosystem’s Zen HQ platform acts as the base to a building management system — enabling scheduling, lockouts, monitoring and alerts — while the Zen LOOP software overlays algorithms that anticipate peak events and additional contextual overrides to save even more money.
To cut demand charges, getting ahead of maintenance issues is key. When strained or overworked, a device often uses energy excessively and can therefore be the reason for a high demand charge. Commercial energy system operators can use monitoring and predictive analytics and set maintenance schedules and alerts into energy management platforms to get ahead of maintenance issues.
Another key pattern in high demand charges is human behavior. For example, seasonal changes in temperature can influence people to tamper with HVAC equipment excessively and therefore lead to higher demand charges. Having systems in place like partial enabler features that grant only partial control helps limit human behavior’s impact on demand charges. These features, for example, allow employees to make adjustments to a thermostat within a set temperature range or limit the number of changes each energy manager can make.
To respond to a demand response event, commercial entities can perform actions like turning off non-critical devices such as decorative lighting, adjusting temperature set points or turning off pool pumps, for example in hotels. They can also automate these actions with software based on time-of-use pricing.
Energy management systems are moving from basic demand response to algorithmic demand response, which leverages large sets of data and intelligence for predictive analytics and even better economics. For example, leveraging advanced weather tracking and forecasting technologies, energy management systems can now predict extremely hot days before they occur. These systems can therefore anticipate when there will be grid strains and demand response events. Software and demand management can ensure the commercial customer has resources available to shed, for example, by pre-cooling a room ahead of an anticipated peak event.
Understanding demand management incentives and implementing AI-enabled actions are turnkey ways to help small to midsized businesses cut costs. For businesses with multiple HVAC devices or buildings across a geographic area, these software-enabled, energy-saving actions can have a big impact on the bottom line and help utilities ensure the safe and reliable operation of the grid in real time. Additionally, enabling greater and more flexible demand management at scale can help renewable energy providers manage the intermittency of solar and wind on the grid and enable greater renewable energy adoption.
James McPhail is CEO of Zen Ecosystems. Having worked with over 3,000 commercial sites managing peak demand charges and demand response, Zen Ecosystems can identify patterns and apply lessons learned from orchestrating energy savings for the commercial segment at scale.
Want to read more about Energy Management topics?
Check out all the latest facility management news related to Energy Management.